With the rapid integration of AI technology into products, designing for AI has become a crucial element of UX design process. To stay ahead of the curve and build effective, intuitive, and solutions, product teams need to adherence to new human-AI interaction principles.
In this article, we’ll help you embrace those new principles by walking you through 12 expert tips for designing for AI. By the end of this article, you will have the knowledge you need to create better AI user experiences.
- Why is designing for AI important?
- Designing for AI: 12 Expert Tips for Human-Centered Design
- 1. Design with usability in mind from the start
- 2. Understand the user’s needs and expectations
- 3. Involve project stakeholders in the AI UX planning
- 4. Be clear about what the AI system can and can’t do
- 5. Aim to deliver contextually relevant information to users
- 6. Proactively plan for the AI system to fail
- 7. Take into account the impact of bias on AI systems
- 8. Provide global controls for AI behavior and permissions
- 9. Be explicit about where human review and intervention is needed
- 10. Make known the data privacy and security policies
- 11. Foster a culture of thoughtful and continuous improvement
- 12. Utilize the latest industry AI design patterns
- Conclusion - designing for artificial intelligence technology
Why is designing for AI important?
The underlying technologies behind AI have been around for many years, but it was the ChatGPT user experience that brought it to the masses and set a record as the fastest-growing software tool, with over 100 million users and counting.
This novel way to interact with AI initiated by ChatGPT has sparked the need for reliable AI-specific design principles that provide:
- Improved usability: Design-focused AI systems are more user-friendly, accessible, and adaptable, catering to a broad spectrum of user needs.
- Trust building and transparency: Effective design aids in demystifying artificial intelligence. By clearly communicating how artificial intelligence tools work, it fosters user trust and sets explicit expectations, reducing the risk of misunderstandings that could lead to significant problems.
- Bias reduction: A considered design approach plays a pivotal role in identifying and mitigating biases within AI tools. This not only ensures fairness but also helps prevent incidents that could harm the system's reputation or usability.
- Personalized experiences: Effective design enables superior personalization, making artificial intelligence products feel more tailored to individual users, thereby increasing engagement and satisfaction.
- Ethical considerations: Design is integral in ensuring AI systems respect critical aspects like user privacy and consent. By embedding ethical considerations into the design, we can avoid mishaps that could lead to user distrust or legal complications.
By integrating these design principles directly into the development process and creative workflow, we can address the challenges posed by the diverse and rapidly evolving landscape of AI design.
Designing for AI: 12 Expert Tips for Human-Centered Design
It’s no secret that AI projects are complex, often involving a multi disciplinary team, both business and technical stakeholders, and at times, even expert AI consultants. It’s not easy to integrate new practices into an already complex development process. The first step to creating more human-centered AI designs is to simply acknowledge this fact.
While project complexity can be a barrier to integrating AI design best practices into your workflows, over time you’ll likely find that embracing a human-centered approach to AI design will help produce more reliable and useful AI systems that achieve greater ROI in software development.
In this section we’ll provide 12 expert tips to help your team take their first steps towards developing more human-centered AI designs.
Design with usability in mind from the start
Designing AI systems with usability as a primary consideration from the inception is crucial in today's world. The success story of ChatGPT, while still a work in progress, is a testament to this approach.
By merging the transformative capabilities of LLMs with an effective and straightforward UX/UI, the product managed to resonate with a vast user base. The message is clear: instead of developing complex, inaccessible programs, we can unlock the power of AI through simplicity.
To design with usability in mind from the start, the AI team should:
- Prioritize user needs: Focus on understanding and addressing user preferences and requirements, ensuring your AI system simplifies tasks and enhances the user experience.
- Create intuitive interfaces: Develop user interfaces that are easy to navigate and require minimal prior knowledge about AI, fostering widespread adoption.
- Incorporate feedback channels: Establish mechanisms for users to offer feedback on system performance, enabling continuous improvement based on real user experiences.
- Use accessible language: Communicate with users using clear, non-technical language to ensure the system is easily understood by all.
- Implement regular updates: Stay committed to user satisfaction by frequently updating and refining the AI system based on changing needs and user feedback.
Real Example: ChatGPT emerged as the leader in the AI space not because they provided a particularly novel use of AI, but rather because they prioritized usability and accessibility to the masses. They looked at the bigger picture and developed a simple interface that allows users to intuitively know how to interact with what, up until that point, had felt like an inaccessible technology only for a select few.
Understand the user’s needs and expectations
Understanding the user's needs and expectations is an integral part of designing AI products and systems, and it's something that needs to be considered upfront. This is not a task that can be superficially addressed at the user interface (UI) level or added as an afterthought during the later stages of the development process.
If user needs and expectations are not built into the product specifications from the beginning, it can be extremely challenging, if not impossible, to retrofit the product to meet those needs effectively.
To ensure your AI product is truly user-centric, the AI team should:
- Identify users and stakeholders: Know who will be using your product and who will be affected by its implementation. This could be a specific demographic, industry, or internal team who uses computer systems that will be replaced by the AI tool.
- Engage with users and stakeholders: Conduct in-depth interviews or workshops. Understand their needs, pain points, desires, and expectations in relation to your AI product.
- Use surveys and questionnaires: Complement your qualitative findings with quantitative data. Surveys can help you reach a larger audience and identify trends or common themes.
- Observe user behavior: If possible, observe users in their natural environment. Identify processes or workflow that normally require human intelligence, but will be replaced with AI.
- Create user personas and use cases: Based on your research, create detailed user personas and use cases. These should reflect users' goals, motivations, behaviors, and potential interactions with the AI system.
Real example: Microsoft’s Copilot is an AI assistant built right into the Microsoft 365 apps. Providing real value to the users required the product team to have a deep understanding of not only the users’ expectations but also how they use the existing Microsoft 365 software tool, which in this case was Microsoft Word. With adequate user research, they were able to build in features like what you see below, where the Copilot AI is suggesting relevant documents based on the user’s prompt.
Involve project stakeholders in the AI UX planning
Designing AI should never be an isolated process. Establishing a robust foundation for effective AI design is rooted in mindful UX planning. It's essential to proactively involve stakeholders who can contribute diverse perspectives and insights, and provide valuable input on project constraints and priorities.
Including these key voices in the planning phase guarantees that the final product is not only user-centric, but also harmonizes with business objectives and adheres to all legal, ethical, and technical guidelines. This multifaceted approach ensures a well-rounded, effective AI design that meets both user and business expectations.
To involve stakeholders in the AI UX planning, the AI team should:
- Identify and understand stakeholders: Determine who has an interest in your artificial intelligence project, from internal teams to external users. Conduct interviews to understand their needs, expectations, and constraints. Their insights can shape the direction and constraints of the AI system, ensuring it aligns with business goals and user needs.
- Engage in co-creation workshops: Foster active involvement in the AI tool design process through co-creation workshops using collaborative design tools like Miro. This collaboration allows stakeholders to contribute their ideas and provide feedback on the project’s creative assets, leading to innovative solutions that are more likely to gain their buy-in and support.
- Validate plans and foster ongoing engagement: Once your AI UX plan is drafted, validate it with stakeholders. Then, keep them involved throughout the project lifecycle. Regular updates, feedback sessions, and check-ins ensure alignment, prevent misunderstandings, and maintain stakeholder support.
Real Example: For a team developing a hospital AI system that helps doctors diagnose illnesses, key stakeholders may include doctors, nurses, IT staff, and hospital administrators.The AI development team could host co-creation workshops to foster collaborative design, such as a user-friendly interface that meets the needs of doctors who speak multiple languages, efficient data processing for IT staff, and privacy controls for hospital administrators. If not all stakeholders are available to meet in person, the project team could alternatively:
- Set up video interviews to capture stakeholder input
- Send out surveys to get input on specific design decisions
- Conduct A/B testing with key stakeholders to get a sense for user preferences
Be clear about what the AI system can and can’t do
Now that we’ve covered a couple of tips for initiating and managing your AI project to prioritize design, let’s switch gears and get into some more specifics about the design of the AI system itself.
One of the most crucial functions of AI machines is transparency. Being transparent with the end users about the capabilities and limitations of an AI system is crucial for user satisfaction and trust. Clear communication can help set realistic expectations, aid users in knowing how to perform tasks with the AI, prevent misunderstandings and help avoid system misuse.
To achieve AI transparency, the AI team should:
- Ensure clear UI communication: Employ concise language in the user interface to outline the system's capabilities and limitations. This can be via tooltips, help sections, or system responses. Teams can also collaborate with the product and marketing teams to ensure that any marketing campaigns accurately portray the functionality of the system.
- Prioritize user onboarding and feedback: Include information about the AI's abilities and limits during user onboarding using interactive tutorials or guided tours. If the AI can't perform a requested action, provide clear feedback and, if possible, suggest an alternative.
- Provide continuous updates: Update communications as the AI evolves, so users always understand the system's capabilities. This could include email notifications to users or in-application notifications.
Read More:
Real Example: Microsoft’s Copilot built right into PowerPoint makes it clear what it can and can’t do by exposing system controls that prompt the user to “adjust” and “regenerate” the AI-created slides by providing example inputs. This is a great way to bridge the gap between the AI system and users, as it provides concrete ways for the user to try interacting with the system.
Aim to deliver contextually relevant information to users
The value of AI really lies in providing specific, contextually relevant support to the user. The more the system knows about the user and their work, the more value it should be able to provide. Designers working on AI systems should be aiming to deliver contextually relevant information to users. Doing so significantly elevates the user experience by supplying pertinent details tailored to the user's current situation or task, rather than generic, high level support. This approach also reduces the cognitive load on users, enhances user satisfaction, and can boost engagement by avoiding irrelevant responses or suggestions.
To deliver contextually relevant information, the AI team should:
- Conduct adequate user research: Providing contextually relevant information relies on a deep understanding of how users interact with the software, what their current workflows look like, and what information they might find helpful from AI. Refer to tip #1 above for more details on this.
- Ensure collaboration between the UI designers and developers: Designing an AI system to be responsive to the user's context requires not just good front-end design, but also thoughtful use of data like location, usage patterns, or user inputs. There should be intentional collaboration between the front-end and back-end development teams to achieve success in this area.
- Provide personalized assistance: Utilize machine learning algorithms to personalize responses based on the user's context.
Real Example: Microsoft’s Business Chat shows users contextually relevant information that’s based on the user’s workflow, documents, calendar, and more. This type of AI design can transform how someone works - creating a true “smart” assistant that can reduce manual work and help the user get more done, faster.
Proactively plan for the AI system to fail
Acknowledging from the outset that even the most advanced AI can encounter errors or unexpected scenarios is critical. This mindset equips the AI team to construct robust responses for when failures occur. During such instances, users should be promptly informed to maintain trust and minimize frustration.
It's important to remember that the repercussions of AI failures can span from mild user irritation to severe physical harm or even loss of life. Because of this, it’s imperative that the AI team properly scopes out the risks early in the project. This way you can build in adequate safeguards and error tolerance.
To plan for failure, the AI team should:
- Anticipate errors: Early in the development process, brainstorm potential technical and user-centric failures. Consider the consequences of errors, specifically false positives and negatives, and gauge their impact on the user.
- Build in a default for unexpected behavior: You won’t be able to anticipate every potential failure. Users interacting with AI tools at scale will trigger unexpected paths. Build in default behavior for when the AI fails or reaches an unexpected path they don't know how to handle. Design for graceful degradation: When the system fails, it should still retain some functionality or give useful feedback rather than just crash or show an error message.
- Incorporate clear feedback and remediation methods: If an action can't be performed, the system should explain why and suggest alternatives. Plan remediation methods such as setting user expectations, providing manual controls, and offering high-quality customer support.
Real Example: A great way to handle simple AI failures on the UI side is to have the AI gracefully address the lack of understanding or failure and provide an alternative solution. This is especially important for AI tools that are being used by external users, as customer satisfaction can degrade quickly if a user isn’t provided with a resolution to their problem when the AI fails.
Take into account the impact of bias on AI systems
Bias in artificial intelligence can be understood as the system providing skewed or prejudiced outcomes. This usually happens when the data used to train the system is biased itself. Bias can manifest in numerous ways - it could be based on race, gender, age, or any other demographic or personal characteristic.
And, the key thing to know here is that every AI system will have some amount of bias. It’s unavoidable. Your job is to ensure that you’re aware of the potential biases of your system and take steps to minimize this bias.
To minimize bias, the AI team should:
- Understand the source of bias: Begin by understanding where bias in AI can come from, such as:
- Bias in data collection: This is the most common form of bias, where the data used to train the AI model is not representative of the population it's intended to serve. For instance, if a facial recognition system is trained mostly on light-skinned and male faces, it may perform poorly when identifying women and people with darker skin.
- Bias in algorithm design: Sometimes, the algorithm itself could be designed in a way that leads to biased outcomes. This usually occurs when the algorithm is designed to prioritize certain features over others. For example, a hiring algorithm might prioritize candidates with certain types of experiences or from certain schools, inadvertently disadvantaging equally qualified candidates who don't fit those criteria.
- Bias in interpretation: Even if the data and algorithm are unbiased, the interpretation of the AI's output could still introduce bias. For example, if a system is designed to predict the likelihood of criminal reoffending, and its outputs are used without considering social or economic factors, it could lead to unfair sentencing or parole decisions.
- Diversify training data: Aim to use a diverse and representative dataset for training the AI system. This can help minimize the bias in the AI's outputs. Be mindful of any missing or underrepresented groups in your data.
- Utilize bias detection and mitigation techniques: Use statistical techniques and AI bias detection tools to identify and reduce bias in your AI system. This could involve techniques like fairness metrics, bias audits, or adversarial testing.
- Prioritize diversity on the design and testing team: Involve a diverse group of users in the design and testing process. This can help you uncover potential biases and ensure the system works well for all users.
- Transparency and Explainability: Be transparent about the potential biases in your AI system and how you're addressing them. If possible, design the system to explain its decisions, which can help users understand any potential bias in those decisions.
Real Example: A design team creating facial recognition software using computer vision technology for a security company is aware that the data available to train their AI model is not representative of all skin colors and features. To address this bias in the data, the team could:
- Take proactive steps to include a more diverse dataset, including sourcing real facial image data or using image generation to create more diverse data
- Involve a diverse group of users in the testing process to help ensure the appropriate level of new data has been incorporated into the model and product design
- Integrate design elements into the AI system that explicitly address any remaining biases in the system
Provide global controls for AI behavior and permissions
Providing global controls for AI behavior and permissions is essential for businesses developing user-centric AI systems. It allows users to dictate the AI's behavior and data monitoring on a system-wide scale. This includes control over specific AI settings or parameters and limiting the types of information the AI collects, thereby enhancing user trust and enabling more personalized experiences for individual users and businesses.
To provide users global controls, the AI team should:
- Construct intuitive interfaces: Develop a user-friendly settings interface that allows users to effortlessly modify AI behavior and permissions.
- Promote transparency: Provide clear explanations of each control, detailing its effects and the advantages of granting data access, to ensure the user fully understands their choices.
- Prioritize privacy: Implement a privacy-first approach, necessitating explicit user consent before accessing sensitive data or functionalities.
- Encourage regular reviews: Prompt users to frequently reassess their settings, taking into account any updates in the AI system or changes in their personal preferences.
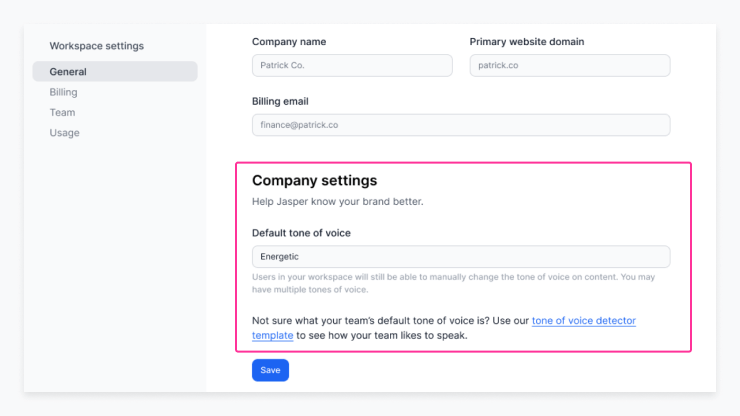
Be explicit about where human review and intervention is needed
As you’ve seen, much of AI design work involves providing the human interacting with the AI sufficient information and control of the system. This next tip follows that same path. Just as you want to inform users of the potential uses for your AI tool, you also want to guide them to provide input at the right times during use.
Being explicit about where human review and intervention is needed is a crucial strategy in creating user-friendly and reliable AI systems. AI is not infallible; there are cases where human oversight is beneficial, such as when the system encounters uncertainty, requires user feedback, or experiences a failure. Being explicit about these instances can foster user trust, improve system reliability, and create a more collaborative relationship between the user and the AI.
To be more explicit about where human review is required, the AI team should:
- Address system uncertainty: Design the AI to seek human input when facing ambiguous situations or uncertain decisions, using methods such as confirmation pop-ups or detailed input interfaces.
- Facilitate user feedback: Implement mechanisms for users to offer feedback on the AI's behavior through ratings, comments, or direct interactions, which can greatly enhance the system's performance over time.
- Prepare for failures: Devise plans to address AI system failures, incorporating clear avenues for human intervention, detailed error messages, and robust user support systems.
- Maintain transparency: Communicate openly with users about when and why human review or intervention may be required, sharing this information through the user interface, documentation, or onboarding process.
- Embrace continuous learning: Utilize human review and intervention instances as learning opportunities for the AI, allowing the system to evolve and reduce the need for human involvement in the future.
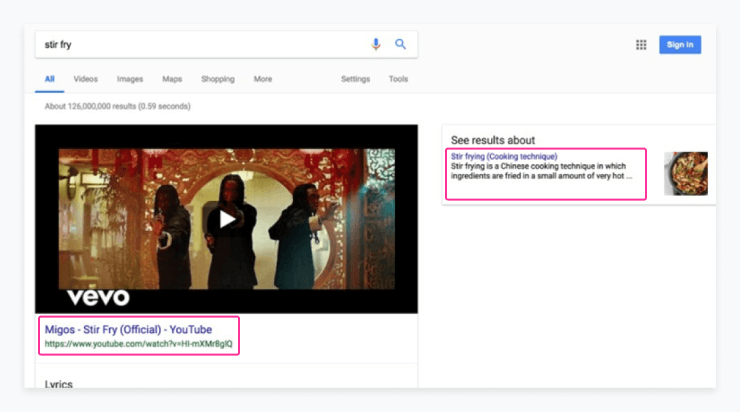
Make known the data privacy and security policies
Making known the data privacy and security policies is a key part of responsible and trustworthy AI design. Clear communication about how user data is handled and protected helps build user trust and helps companies comply with data protection laws and regulations.
To achieve greater privacy and security transparency, the AI team should:
- Craft transparent policies: Develop clear, concise, and transparent privacy and security policies, avoiding technical jargon and legalese to ensure comprehensibility for all users.
- Ensure accessibility: Position these policies within easy reach in the AI system, such as in the settings or help menu, and consider sharing policy highlights during the onboarding process.
- Prioritize user consent: Secure explicit user consent for data collection and usage at all times, and provide a straightforward method for users to adjust their consent settings.
- Commit to regular updates: Amend your policies in line with the evolution of your AI system or changes in data protection laws and regulations, and keep users informed about these updates.
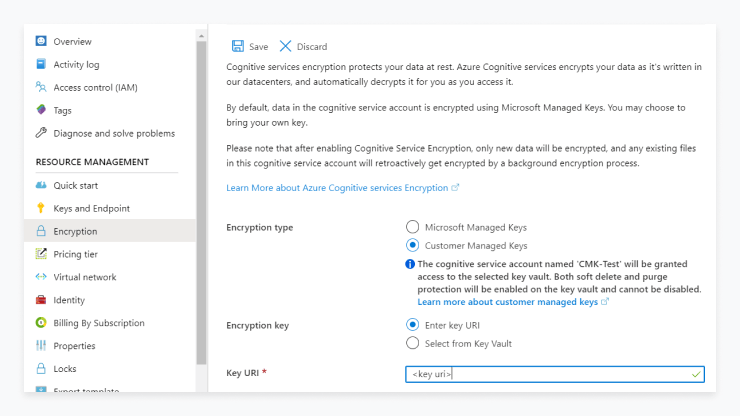
Foster a culture of thoughtful and continuous improvement
Cultivating a culture of mindful and perpetual improvement isn't just advantageous in AI development—it's absolutely vital. With the evolution of AI technology, team expansion, and shifting business requirements, a foundation of continuous learning and adaptability becomes a cornerstone for success for teams committed to long-term AI investments.
This approach guarantees that your AI system not only fulfills current demands but is also primed to tackle future challenges and seize emerging opportunities.
To foster a culture of continuous learning, the AI team should:
- Encourage feedback: Create a culture where feedback is not only welcomed but also actively sought. This stimulates dialogue, fosters ownership, and seeds a collective drive for enhancement. As the team and business grow, these open channels of communication become vital to maintain alignment and shared vision.
- Facilitate continuous learning: Encourage team members to continually learn and stay abreast of AI advancements. This fuels an organizational growth mindset that embraces change and keeps the AI system on the cutting edge of technology, even as the landscape evolves.
- Conduct regular reviews: Conduct regular performance reviews to identify areas for improvement. In a rapidly evolving tech landscape, these proactive assessments can help the AI system stay relevant and effective, adapting to both internal changes and external market dynamics.
Real Example: A team developing an AI-powered customer service chatbot can foster a culture of continuous improvement by:
- Proactively learn about emerging technologies that could enhance the chatbot's performance,
- Encouraging team members to share feedback about the chatbot's performance by sending out surveys through tools like Typeform.
- Gather detailed user input by employing a tool like usersnap that allows users to tag specific areas of the software that they want to comment on.
Utilize the latest industry AI design patterns
Utilizing the latest industry AI design patterns is crucial when developing AI systems. It ensures that your system benefits from the collective knowledge and experience of the AI community, adheres to best practices, and remains competitive in an ever-evolving technological landscape.
Here's how a team can effectively utilize the latest industry AI design patterns:
- Stay informed: Keep up to date with the latest trends, research, and advancements in AI design by following industry publications, attending conferences, and engaging with AI communities. This helps you stay aware of new design patterns and methodologies.
- Collaborate and learn: Actively engage with other AI professionals, both within and outside your organization. Sharing experiences and insights can foster learning and help you stay informed about the latest design patterns and best practices.
- Adapt and innovate: As you learn about new AI design patterns, evaluate their applicability to your system. Be open to adopting and adapting these patterns to improve your system's performance, usability, and user experience.
- Train your team: Encourage team members to participate in training programs and workshops focused on AI design patterns. This builds a shared understanding of the latest design methodologies and ensures that the team stays current with industry standards.
Read more about AI design patterns:
Conclusion - designing for artificial intelligence technology
The rising prevalence of AI underscores the importance of human-centered design more than ever. By embracing the tips shared in this article, you can ensure that your AI systems are intuitive, transparent, fair, and highly personalized, thereby providing an enhanced user experience.
Remember, the path to successful AI design is one of continuous learning and improvement. It may seem complex at first, but with commitment and a user-centric approach, your team can create AI systems that not only meet current demands but are also adaptable for future challenges and opportunities.
If you're looking for help developing an AI application, consider our AI development services. Our team can help you put together a comprehensive AI strategy and guide you through the development process. With our software development consulting services, you can leverage the power of AI models to build a market-ready product that stands out from the competition or increase efficiency and boost productivity with a custom AI-powered application.