Finance teams deal with vast amounts of data, making it a natural fit for generative AI, which has the power to sift through data and other documents, provide contextual awareness, human-like decision-making capabilities, and even generate original content and insights. In fact, McKinsey estimates that generative AI has the potential to deliver between $200 and $340 billion in value to the banking industry alone.
The finance services sector is clearly not sitting on the sidelines of the AI revolution; they are embracing generative AI and actively piloting innovative generative AI initiatives across departments. And in this article we’ll take a closer look at 10 real examples of finance companies doing just that to inspire your own innovation in generative AI in finance services.
Challenges with adopting generative AI
Before we dive into the use cases of generative artificial intelligence in the finance sector, we need to understand the challenges companies are up against. While the barriers to experimentation are relatively low for Gen AI initiatives compared to previous technologies like robotic process automation and process mining, the path to successful implementation is not without its hurdles. Some of the most critical challenges the financial services industry faces when adopting generative AI are:
- Data accuracy: Generative AI tools have the potential to produce outputs that, while highly convincing, may not always be precise or entirely reliable, especially in complex or nuanced scenarios.
- Leaks of proprietary data: Using public cloud services for training generative AI models poses risks of data breaches, exposing sensitive financial information.
- Governance model: The absence of a robust governance framework to validate AI outputs due to lack of contextual awareness and real-time information.
- Hallucinations: The risk of generative AI producing highly convincing but incorrect responses, requiring stringent checks and balances.
Addressing these challenges involves a combination of technological advancements, strategic planning, stringent data security and governance measures to ensure that the adoption of generative AI in finance functions is both effective and secure.
10 proven use cases of generative AI in finance
Despite the challenges the finance sector faces in fully embracing generative AI, many of the top players in this space have already begun aggressively investing in this technology. In this section we’ll zoom in to 10 proven use cases to demonstrate how financial services companies are overcoming the core challenges associated with Gen AI and are using it to revolutionize their back office processes, customer engagement models, and their very operating models.
Data analytics
In the data-intensive world of finance, generative artificial intelligence (GenAI) is playing a pivotal role in reshaping the way finance teams operate. By leveraging GenAI, teams are significantly reducing manual labor, streamlining processes that were once time-consuming and prone to human error. The technology's ability to process and analyze vast amounts of data at unprecedented speeds allows finance professionals to focus on more strategic tasks that require human insight and decision-making capabilities.
This integration of GenAI in finance is not only enhancing existing processes but also creating new opportunities for innovation and efficiency in areas such as:
- AI-assisted financial analysis: AI-powered trading algorithms analyze vast amounts of market data, identifying trends, anomalies, and emerging opportunities. This real-time analysis helps investors and traders make informed decisions, mitigate risks, and capitalize on market fluctuations.
- Understanding customer preferences: Through the analysis of customer data, Generative AI can uncover profound insights into the preferences and behaviors of customers, facilitating the creation of tailored marketing approaches and enhancements in customer service.
- Anomaly detection: AI algorithms are adept at spotting atypical patterns or irregularities within extensive datasets, potentially signaling security compromises or fraudulent actions.
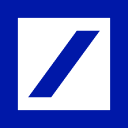
INDUSTRY EXAMPLE:
Deutsche Bank is testing Google's generative AI and large language models (LLMs) at scale to provide new insights to their financial analysts.
Post-trade reconciliation
Another key area where we’re seeing drastic transformation thanks to generative AI is in the back of house processes, specifically post-trade reconciliation. Reconciliation is typically a very complex, time-consuming process, where staff interface across a range of legacy systems. It’s ripe for automation, particularly with the aid of GenAI. Because of the complexity, there are a lot of expensive failures. In fact. The Depository Trust and Clearing Corporation (DTCC) estimates that a trade settlement failure rate of just 2% costs firms up to $3 billion globally.
Gen AI can be used to review data, detect anomalies, and make recommendations for human review. And when you pair this with tools that offer ML or predictive analytics, you can automate the process, identify trends and patterns in the data and apply those to future reconciliations.
The main benefit of applying AI and machine learning to reconciliation processes is the significant reduction or complete removal of manual tasks, leading to substantial savings in time and money. Furthermore, it decreases the likelihood of mistakes that are common in manual reconciliations, thereby reducing financial discrepancies and losses.
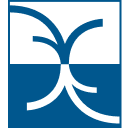
INDUSTRY EXAMPLE:
OpsGPT by Broadridge is a GenAI- and LLM-powered application — a co-pilot for operations users, analysts, and management teams to better manage the post-trade lifecycle. The OpsGPT tool:
- Reduces research time to identify root cause of fails
- Accelerates research of next best action and integrates workflows to resolve the fail
- Prioritizes key risk items
- Provides management insights to better manage workflows and teams through dashboards
Compliance and risk management
Financial institutions face high stakes when it comes to managing security risks and compliance with local and federal regulations. To enhance operational efficiency, ensure regulatory adherence, and proactively mitigate risks, these institutions are increasingly leveraging generative AI. This technology excels in analyzing vast data sets more efficiently and accurately than human capabilities allow, identifying patterns and anomalies indicative of risk or fraudulent activities, and ensuring compliance with regulatory mandates more effectively.
There’s a wide range of potential applications of Gen AI within the compliance and risk management space, including:
- AI-Powered chatbots for compliance queries: Deploy AI chatbots and virtual assistants to address routine compliance questions from both employees and customers, relieving the workload on compliance staff.
- Regulatory document analysis: Utilize generative AI for rapid regulatory and financial document search, analysis, and summarization, helping compliance teams stay abreast of changing regulations.
- Automated compliance reporting: Employ generative AI to draft compliance reports by analyzing financial data from compliance monitoring systems, streamlining the reporting process.
- Risk identification: Apply generative AI to scrutinize customer feedback and social media for potential conduct risks and compliance issues, enhancing risk management.
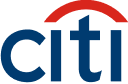
INDUSTRY EXAMPLE:
Citi used AI to analyze 1,089 pages of new capital rules on the U.S. banking sector. The bank’s risk and compliance team used the technology to assess the impact of the plans, which will determine how much capital the lender has to set aside to guard against future losses.
Financial reporting
Following the 2008 global financial crisis, regulators imposed stringent reporting mandates on financial institutions to promote operational transparency. These new requirements led to a significant increase in both the volume and complexity of data that needed to be frequently submitted to regulators. The primary challenge for financial institutions has been the labor-intensive process of deciphering these requirements, converting regulatory mandates into actionable business texts, and then coding these into their systems—a task made even more daunting by the complex organizational structures, and the intricate data and system architectures prevalent within banks.
The advent of generative AI in financial reporting heralds a transformative shift, offering multiple benefits to the sector:
- Efficient data management: AI facilitates the autonomous mining and retrieval of data from varied sources and platforms, significantly reducing the manual effort required to populate reports.
- Process automation: Automating the reporting process from end to end minimizes the need for manual tasks such as mappings, checks, reconciliations, and reviews, thereby saving resources and allowing a shift in focus towards enhancing data quality and analytical depth.
- Regulatory adaptability: The introduction of machine-readable and executable regulatory guidelines simplifies compliance, reducing the time and resources spent on interpreting new or amended regulations and minimizing the risk of misinterpretation.
- Enhanced report quality: Automated checks improve the quality of reports through rigorous data quality assessments, variance analyses, and validation processes.
- Standardization across the industry: AI systems can enforce industry-wide standards for data organization and classification, improving the comparability and analysis of reports by both investors and regulators.
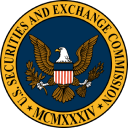
INDUSTRY EXAMPLE:
In the U.S., the SEC has been ratcheting up its use of machine learning since the global financial crisis. The SEC now uses decisions by machine learning models to guide regulators to entities whose behavior required more detailed analysis.
This uses a method called 'keeping the human in the loop', which takes advantage of the power of machine learning but maintains a level of human accountability. All privileged decisions involve a human actor as opposed to autonomously by a machine.
Fraud detection and prevention
Generative AI presents a compelling solution for financial institutions seeking to enhance fraud detection and prevention, particularly due to its capacity to simulate complex fraudulent behaviors and generate synthetic data. This innovative approach not only aids in the development of more accurate and robust fraud detection systems but also ensures the preservation of customer privacy by reducing the dependency on real customer data. The use of Gen AI and advanced machine learning models is revolutionizing the way financial market participants, including payment institutions, tackle Anti-Money Laundering (AML), Countering the Financing of Terrorism (CFT), and fraud.
Key applications of GenAI in fraud detection and prevention include:
- Synthetic data generation: Enhances the training of detection models by simulating sophisticated fraudulent behaviors, improving model accuracy while maintaining user privacy.
- Advanced pattern recognition: Utilizes both supervised and unsupervised machine learning models for detecting fraudulent transactions. Supervised models, like neural networks, learn from historical fraud data to identify similar patterns, while unsupervised models spotlight anomalies, signaling potential risks.
- Real-time transaction monitoring: Enables immediate detection and intervention against fraud by monitoring transactions as they occur, significantly reducing the window for fraudulent activities.
- Adaptive authentication: Adjusts security measures based on transaction risk levels, minimizing obstacles for legitimate transactions while imposing stricter controls on suspicious ones.
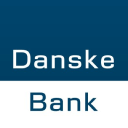
INDUSTRY EXAMPLE:
Danske Bank implemented a modern enterprise analytic solution leveraging AI and was able to:
- Realize a 60% reduction in false positives, with an expectation to reach as high as 80%
- Increase true positives by 50%
- Focus resources on actual cases of fraud
Credit scoring
AI credit scoring transforms traditional credit evaluation methods by leveraging machine learning algorithms to digest a vast array of data points, including unconventional ones, to forecast an applicant's loan repayment capabilities. This approach results in an AI-generated score that offers a richer, more dynamic gauge of creditworthiness, equipping lenders with a deeper, more precise insight into an individual's financial conduct.
However, this innovative approach is not without its hurdles. The primary concerns include the lack of transparency in how machine learning models operate, often described as the "black box" issue, and the risk of perpetuating biases. Platforms like Datrics are at the forefront of addressing these challenges for finance leaders, providing solutions to enhance the fairness and clarity of AI credit scoring.
AI credit scoring, with its advanced machine learning capabilities, significantly revolutionizes the landscape of credit risk evaluation by offering:
- Improved credit risk modeling: Generative AI refines the accuracy of key credit risk metrics such as probability of default (PD), loss given default (LGD), and exposure at default (EAD), by analyzing extensive datasets to uncover complex patterns and relationships that traditional models might not detect, leading to more precise risk assessments.
- Enhanced stress testing: GenAI enables the creation of diverse, challenging scenarios for stress testing, helping financial institutions better prepare for adverse conditions by evaluating how these scenarios could affect their stability and financial health.
- Advanced model validation and bias reduction: By utilizing synthetic data, Gen AI improves the process of model validation, reduces biases, and enhances the transparency of credit scoring models, ensuring they are both equitable and understandable.
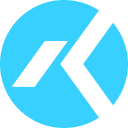
INDUSTRY EXAMPLE:
A UK high street bank leveraged Kortical’s platform to build a machine learning model that beat traditional credit scoring. The model was able to predict credit default better to the point it caught 83% of bad debt not caught by credit score while refusing loans to the same number of customers.
AI powered Robotic Process Automation
Robotic process automation was arguably the last major technology shift in financial services before AI. Through its ability to analyze and interpret intricate data patterns, Generative AI extends automation capabilities to tasks once reliant on human judgment, including:
- Automated document processing: Generative AII elevates RPA capabilities of automating routine tasks and to also summarize financial documents, contracts, and reports. It can understand context, extract relevant information, and even summarize content, thus streamlining document management workflows that were previously labor-intensive.
- Dynamic data validation and cleanup: RPA tasks related to data validation and cleanup can be significantly improved with GenAI. By analyzing patterns and learning from data inconsistencies, GenAI can proactively correct errors, ensuring higher data quality and reliability for financial operations.
- Intelligent customer interaction: RPA can automate basic customer interaction tasks, but GenAI takes this a step further by generating personalized customer responses. This includes drafting tailored emails or chat responses, enhancing customer service automation with a level of personalization and understanding that mirrors human interaction.
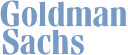
INDUSTRY EXAMPLE:
Goldman Sachs has been conducting tests and pilots to see how AI can help promote efficiency, which has yielded gains of 20% to 40%. Their CIO reported that they are exploring areas ripe for AI-powered automation such as:
- Extracting assets from large data sets
- Generating briefs for clients
- Drafting financial reports
Synthetic data generation
Generative AI excels at generating synthetic data; this data mimics real-world data but does not directly correspond to real individuals. This capability is particularly valuable in the financial sector, where acquiring substantial amounts of data for AI or analytics purposes can be challenging without breaching privacy concerns.
A few key areas where we’re seeing institutions leverage synthetic data generation are:
- Product testing and development: Financial institutions can use synthetic data to test new products and services in a safe and controlled environment. This approach allows for the assessment of potential issues and the refinement of products before they are introduced to the market, ensuring a higher quality and more customer-centric offering.
- Compliance training: Synthetic data can be used to create realistic financial scenarios for compliance training purposes. This enables employees to gain practical experience in identifying and handling compliance-related issues without the risk of using sensitive customer information.
- Build in-house machine learning models: Financial services institutions can use synthetic data generated by Generative AI to build and train in-house machine learning (ML) models without the constraints of limited or sensitive real-world data. This enables the development of robust, predictive models for a wide range of applications, from customer behavior prediction and personalized service offerings to optimizing trading strategies and operational efficiencies.
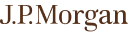
INDUSTRY EXAMPLE:
J.P. Morgan AI Research generates synthetic datasets to accelerate research and model development in the financial services sector.
Personalized banking services
According to a recent Salesforce Research survey 84% of customers emphasize the importance of being treated as individuals, not just numbers, in winning their business. In the finance services and banking industry, teams can leverage generative AI to provide personalized banking services based on customer behaviors, preferences, and financial histories. A few specific personalized services we’re seeing include:
- Dynamic financial planning: Utilizing GenAI to offer tailored financial advice, investment strategies, and savings plans that align with individual goals and risk tolerances.
- Personalized product recommendations: GenAI-driven algorithms analyze a customer's financial situation to suggest the most suitable banking products, such as savings accounts, investment funds, or loans. This targeted approach simplifies the product selection process, significantly enhancing the likelihood of product adoption.
- Customized customer profiles: The creation of detailed customer profiles enables banks to provide customized advice and services. This level of personalization fosters a stronger, more personalized customer-bank relationship, enhancing the overall banking experience.
This approach not only enhances service efficiency but also significantly improves customer satisfaction and engagement, making customers feel valued and increasing their likelihood to engage with more services. Customization deepens trust and connection between customers and their financial institutions by making services more relevant and valuable.
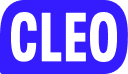
INDUSTRY EXAMPLE:
The Cleo App connects to bank accounts and gives clients proactive advice and information on their finances, including timely nudges to help them stay on top of their spending.
Customer support
AI-powered chatbots and virtual assistants are becoming ubiquitous, revolutionizing the way businesses interact with their customers. The financial sector is no exception, with GenAI playing a pivotal role in transforming customer support services and delivering personalized and efficient assistance. These Gen AI-powered chatbots cater to a broad spectrum of customer needs, from simple account inquiries to providing complex financial advice.
For customer support teams, generative AI offers a range of benefits. First of all, GenAI can significantly accelerate the speed at which customer queries are addressed, providing instant responses that vastly improve upon the delays associated with traditional customer service channels. But it’s not just about speed.
With the ability to analyze and understand individual customer preferences and history, GenAI-driven chatbots can tailor their assistance and advice in real-time, offering support that is not only relevant but also aligned with each customer's unique financial situation. And the adaptive learning capabilities of these AI platforms mean that with every interaction, they enhance their comprehension and problem-solving skills within the finance function.
Financial institutions have a variety of tools at their disposal to develop a custom AI chatbot:
- Chatbase: Chatbase is a robust chatbot platform designed to automate customer support using the power of AI. It offers advanced features for analyzing chatbot interactions, improving responses, and enhancing user experiences.
- Botsonic: Botsonic provides tools for building AI-driven chatbots that can handle complex conversations. It focuses on natural language understanding (NLU) and context-aware responses.
- CustomGPT.ai: CustomGPT.ai allows users to create custom AI models based on the GPT architecture. It’s suitable for enterprises looking to build chatbots with specific domain knowledge.
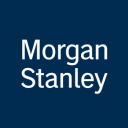
INDUSTRY EXAMPLE:
Morgan Stanley launched Next Best Action, an internally-built, AI-based engine that delivers timely, customized messages to clients and prospects, guided by the Financial Advisor.
Future outlook of generative AI for financial institutions
As we’ve seen, many financial institutions have already begun to invest in generative AI technology. Looking ahead, we expect this trend to grow, moving beyond basic process improvements to fundamentally change how financial services teams operate, interact with customers, and leverage data for better decision-making.
Key opportunities and trends to watch include:
- Enhanced synthetic data usage: As privacy concerns and data regulation continue to grow, the use of synthetic data for training AI models will become more prevalent, enabling the development of powerful AI tools without compromising customer privacy.
- AI in financial education and inclusion: Generative AI could democratize financial knowledge, providing personalized educational content and advice to underserved communities, thereby promoting financial inclusion.
- Expansion of personalized financial products: Leveraging deep insights into customer behavior and preferences, financial institutions will be able to offer bespoke solutions that meet the unique needs of each customer, enhancing satisfaction and loyalty. As the technology advances and new, more specialized Gen AI tools emerge, the level of personalization that will be possible will improve.
Conclusion
Generative AI (GenAI) is transforming the financial services sector by enhancing efficiency, accuracy, and personalization across various functions from data analytics to customer support. Financial institutions embracing GenAI are positioning themselves for significant competitive advantage and innovation. As GenAI evolves, its integration into finance promises not only to optimize current operations but also to redefine the industry's future landscape.
If you’re looking for a partner to help you develop a custom AI solution? Our team will partner with you to build a comprehensive generative AI solution. We'll assist you in selecting the most suitable technologies, seamlessly integrating them into your existing tech stack, and delivering a robust, scalable solution.