In an era where 90% of the world's data was generated in the last two years, and data volumes double every two years, financial institutions face the monumental task of navigating through this deluge of information. In this environment, predictive analytics emerges as a critical tool, transforming vast data into actionable insights for competitive advantage, strategic foresight, and enhanced decision-making.
In this article we explore the top 10 use cases for predictive analytics in finance, demonstrating its power to not only predict future market trends but also to revolutionize investment strategies, risk management, customer engagement, and beyond. We’ll provide tangible resources to help your team embrace predictive analytics and transform data into strategic assets.
- What is predictive analytics in finance?
- 10 Proven Uses Cases of Predictive Analytics in Finance
- 1. Macroeconomic trend reporting
- 2. Earnings predictions
- 3. Stock price returns and volatility predictions
- 4. Optimal portfolio predictions
- 5. Audit and compliance predictions
- 6. Credit risk assessment
- 7. Customer acquisition and attrition prediction
- 8. Customer segmentation and sales prediction
- 9. Credit default prediction
- 10. Financial and credit fraud prediction
- Conclusion
What is predictive analytics in finance?
Predictive analytics encompasses a broad set of techniques, including statistical analysis, modeling, data mining, and advanced machine learning methods, to analyze and interpret current and historical data with the goal of forecasting future events or trends.
Financial institutions are leveraging predictive analytics for a wide range of applications from stock market performance prediction to customer retention forecasting.
Finance companies are increasingly incorporating predictive data analytics into their Decision Support Systems (DSS), which integrate data from both internal information systems (IS) and extensive external databases to provide a comprehensive data foundation for analysis. The diagram below illustrates how this might work.
Recent developments in machine learning have further enhanced the capabilities of predictive analytics in a DSS, introducing a dynamic element of continuous learning and adaptation (as noted in the “learning” module above). This evolution allows predictive analytics models to refine their accuracy over time as they are exposed to new data, leading to more precise and reliable forecasts.
10 Proven Uses Cases of Predictive Analytics in Finance
It’s clear that predictive analytics offers finance organizations a compelling value proposition - new levels of data insights, enhanced decision making, and a competitive advantage. To give you a more concrete idea of how to leverage this technology within your own organization, in this section we’ll dive deeper and look at 10 proven use cases of predictive analytics for finance teams.
Macroeconomic trend reporting
A wide range of financial companies - from banks to investment firms - rely on macroeconomic trends to inform their strategic decisions and manage risk. By analyzing historical data and current economic indicators like GDP growth, inflation, unemployment, and consumer spending, predictive analysis tools can assist institutions in:
- Analyzing macroeconomic indicators: They sift through vast amounts of historical data and scrutinize current economic indicators such as GDP growth, inflation, unemployment rates, and consumer spending. This comprehensive analysis aids in understanding the underlying patterns that drive economic dynamics.
- Forecasting future economic trends: By identifying these patterns, predictive analytics tools enable financial experts to forecast future economic trends with greater precision. This foresight is crucial for anticipating market movements, adjusting investment strategies, and identifying emerging opportunities in a timely manner.
- Developing resilient strategies: Armed with insights from predictive analytics, financial institutions can formulate strategies that are robust against economic fluctuations. Whether facing potential recessions, periods of economic boom, or stagnation, these strategies are designed to safeguard assets and capitalize on growth opportunities, irrespective of the prevailing economic conditions.
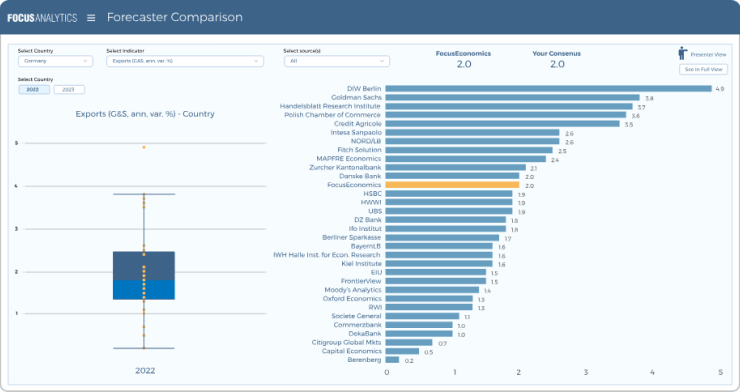
Earnings predictions
Predictive analytics transforms the challenge of forecasting corporate earnings by leveraging advanced computational models. Techniques such as neural networks, genetic algorithms, and support vector machines (SVM) stand out for their ability to handle the complex, idiosyncratic nature of earnings data.
The accuracy and objectivity provided by these models are crucial for financial valuation and decision-making. Research comparing SVM to traditional forecasting methods, such as ARIMA models, underscores SVM's superior performance in quarterly earnings predictions. This shift towards sophisticated models like SVM and genetic algorithms not only bolsters the reliability of earnings forecasts but also aligns with the demand for independent, data-driven insights in predictive analysis, marking a significant advance in the field of predictive analytics.
PRO TIP: To build an earning predictions model, start by accessing data from free or for-pay APIs, such as:
- Financial Modeling Prep (FMP) API: FMP offers various endpoints for financial data, including analyst stock recommendations, company outlook, stock peers, and more. Specifically, their Earnings Calendar API provides upcoming and past earnings announcements for publicly traded companies, along with estimated and actual earnings per share (EPS).
- Benzinga API: Benzinga provides data on future earnings dates and historical results. Their API offers a comprehensive suite of earnings data, which is considered to be of high quality and accuracy. This includes valuable insights into a company’s financial health, growth prospects, and future outcomes.
- Barchart Market Data APIs: Barchart provides an Analyst Estimates API, offering earnings estimate data for over 4,500 rated companies. This data includes consensus estimates, the number of analysts, high and low estimate ranges, growth rate projections, expected report dates, and historical information.
Stock price returns and volatility predictions
Predictive analytics in finance can also be used to forecast future stock price returns and stock volatility. By leveraging vast amounts of historical price data, economic indicators, and even sentiment analysis from news articles and social media, analysts can develop predictive models that estimate future price changes with a certain degree of accuracy.
The prediction of volatility, a measure of the price variation of a financial instrument over time, is particularly crucial for risk management and option pricing strategies. Volatility forecasting models, such as GARCH (Generalized Autoregressive Conditional Heteroskedasticity) and its variants, analyze past price fluctuations to estimate future volatility.
PRO TIP: Keep a close eye on new research and open-source algorithms such as ALERTA-Net, which is an AI-powered model introduced by two South Dakota State University researchers that anticipates stock price movement and stock market volatility trends. It uses a deep neural network that integrates macroeconomic data, search engine data and social media data — the first of its kind to do so.
If you’re looking for a direct access to stock return data to build your own predictive model, consider:
- Alpha Vantage: Free APIs for historical and real-time data, including stock prices, forex, and cryptocurrencies.
- IEX Cloud: Platform that offers a wide range of financial data including stock prices, fundamentals, and market data. It has various plans, including a free tier with limited access and paid subscriptions for more extensive data access.
Optimal portfolio predictions
Predictive analytics plays a critical role in optimizing financial portfolios, offering investors and portfolio managers sophisticated tools to enhance returns while managing risk. Through the analysis of historical market data, financial indicators, and even investor behavior patterns, predictive analytics models can identify potential investment opportunities and forecast future market trends. Specifically, predictive analytics can help in areas such as:
- Asset allocation: Predictive analytics deploys sophisticated models and historical data analysis to craft the optimal mix of assets for each investor’s financial goals and risk appetite. It scrutinizes past market behaviors and economic indicators to recommend a portfolio composition that aligns with the investor's objectives, adjusting dynamically to maintain this alignment over time.
- Diversification: Through the examination of a broad spectrum of investments, predictive analytics facilitates risk mitigation by pinpointing assets that exhibit low correlation with one another. It advises on the inclusion of diverse asset classes, industries, and geographical areas to construct a well-rounded portfolio that aims to enhance returns while minimizing exposure to any single source of risk.
- Rebalancing: By continually assessing portfolio performance against the desired asset distribution, predictive analytics identifies when a portfolio may be drifting from its target allocation. It signals the need for rebalancing, recommending specific trades to realign the portfolio with the investor’s strategic goals and risk tolerance,
PRO TIP: Choose an API or predictive analytics tool based on your needs:
- Portfolio Optimizer is a good choice for developers and teams who are interested in building a custom portfolio optimization solution via an API that is grounded in Nobel Prize-winning science.
- Boosted.ai is best for financial advisors and institutional investors looking for an all-in-one platform to access an AI analyst assistant along with other tools such as investment idea generation and process automation.
- Portfolio Visualizer is geared towards companies looking for a more traditional investment optimization platform experience that can guide predictive analytics and simulations.
Audit and compliance predictions
The compliance landscape within financial institutions is transforming, with a shift from traditional manual processes to the adoption of machine learning (ML) and intelligent automation. This evolution, driven by the need for enhanced risk management and operational cost reductions, leverages historical data and predictive modeling to proactively identify and mitigate compliance risks.
At the core of this transformation are two key strategies:
- Enhanced risk assessment: Utilizing past compliance data, predictive analytics identifies patterns that spotlight areas prone to non-compliance, enabling strategic prioritization of audit and compliance efforts.
- Proactive regulatory compliance: By analyzing regulatory compliance trends, predictive analytics forecasts potential breaches, facilitating the implementation of preemptive measures to avert violations. Integral to this strategy is the role of cash flow forecasting in maintaining financial stability and regulatory compliance.
This approach not only aids in avoiding non-compliance and potential financial penalties but also protects the institution's reputation and ensures regulatory mandates are met.
PRO TIP: Try Akkio, a no-code platform designed to help businesses integrate ML and predictive analytics into their existing solutions. It offers a targeted solution specifically for compliance applications. With Akkio, you’ll be able to:
- Create predictive AI models without needing extensive ML knowledge.
- Set up ML models quickly, automate routine compliance tasks, and have human experts monitor these models to flag issues and focus on higher-risk projects.
- Connect multiple datasets and integrate Akkio with your existing data collection tools, like Salesforce, Snowflake, Google Big Query, Google Sheets, HubSpot, and Zapier.
Credit risk assessment
Predictive analytics and artificial intelligence (AI) credit scoring are revolutionizing the way financial institutions assess credit risk. By analyzing a diverse array of data sources—ranging from credit histories and transaction records to social media activities—these technologies uncover patterns and correlations beyond the reach of traditional credit scoring methods.
This dynamic approach not only identifies borrowers with high credit potential for targeted programs but also improves risk management by excluding higher-risk individuals. The integration of AI and predictive analytics into creditworthiness assessment processes thus promises more accurate profit forecasts, informed lending decisions, and a more inclusive and efficient credit system.
PRO TIP: Whether you want an out-of-the-box AI-powered predictive analytics tool for credit risk assessment or you want to build your own with available data sets, there are resources available. If you’re looking for a APIs to build a custom credit risk assessment model, consider:
- Kaggle, which offers a variety of credit risk data sets as well as hosts competitions to build cutting-edge credit risk predictors.
- FinPricing Credit Spread Curve Data API, which provides credit spread curves for various sectors and ratings.
Or if you’re after an out-of-the-box platform experience, consider:
- H2O.ai, a platform providing an AI-driven approach to credit scoring by optimizing credit portfolios, verifying proof of income, and automating credit decisions. It outperforms traditional scorecards in both consumer and business lending.
- Datrics, a platform to perform precise credit risk assessments and credit scoring based on masses of data, enabling accurate eligibility forecasting and smart borrower ratings.
Customer acquisition and attrition prediction
Predictive analytics is not just being used by financial analysts and leadership teams, it’s also being used by marketing and product teams within financial organizations to transform customer acquisition and retention strategies. By analyzing demographic details, buying habits, and online interactions, predictive analytics models can be used to:
- Pinpoint ideal customer traits: Utilize predictive analytics to identify the defining characteristics of potential financial services customers, accurately forecasting those most likely to become loyal clients.
- Estimate customer lifetime value (CLV): Employ historical data and machine learning techniques to predict future behaviors and accurately estimate the lifetime value of customers, aiding in strategic resource allocation.
- Detect early signs of customer churn: Analyze customer engagement metrics, such as decreased usage or payment delays, to proactively identify customers at risk of churn, enabling preemptive retention strategies.
PRO TIP: There are many predictive analytics platforms available to kickstart customer intelligence work.
- If you’re looking for a platform specifically designed for FinTech companies that can help you improve your customer acquisition strategy, consider Success.ai.
- If you’re looking to focus on reducing your customer churn, consider Braze.
- If you’re looking to build a customer churn model based on your company data - all without the need for any data science or programming skills - consider Userpilot.
Customer segmentation and sales prediction
Customer segmentation is a process of dividing customers into groups based on shared characteristics, such as demographics, behavior, or preferences. By doing so, banks and other financial institutions can tailor their marketing and sales strategies to better meet the needs and expectations of each group. The practice of customer segmentation has been around for decades, but with the advent of predictive analytics, it has become even more powerful and effective.
Mapping customer interactions—such as clicks, signups, and video views—creates a rich behavioral dataset for predictive algorithms. This detailed data analysis fine-tunes customer segmentation and boosts the effectiveness of marketing and sales strategies, ensuring they're closely aligned with the diverse needs and preferences of different customer groups.
It’s worth noting that financial organizations with over 100,000 monthly active users benefit most from predictive customer analytics, thanks to the large data volumes that enable more accurate forecasts.
PRO TIP: Try using an AI-powered predictive analytics tool to optimize your customer segmentation and sales prediction strategy:
- Peak AI is an AI-powered customer segmentation tool that enables personalized experiences to win new customers and delight existing ones.
- Amplitude uses predictive analytics to develop smart customer segments for better informed marketing strategies.
- Twilio segment predicts customer behavior and builds targeted audiences for more personalized marketing campaigns that lower costs and increase conversions.
Credit default prediction
Credit default prediction is a more specific aspect of credit risk assessment focused on identifying the likelihood of a borrower failing to repay their loan — in other words, defaulting. This process utilizes statistical predictive models and machine learning algorithms to analyze data and identify patterns that indicate a higher risk of default.
The effectiveness of AI/ML-based loan default prediction models is significant, transforming risk management strategies within the financial sector. This methodology goes beyond traditional credit evaluation techniques by incorporating a wide array of variables, including borrowers' financial history, spending patterns, and even non-financial indicators that could hint at their future financial behavior.
Financial and credit fraud prediction
By harnessing vast amounts of transactional data, alongside behavioral patterns of customers, predictive analytics models can identify anomalies that deviate from the norm, signaling potential fraud. These models are trained on historical fraud data, allowing them to learn and recognize the subtle signs of fraudulent transactions, whether they're originating from credit card use, loan applications, or digital payment platforms.
By predicting fraud risks with high accuracy, banks can implement targeted security protocols for high-risk transactions, thereby minimizing the inconvenience to legitimate customers and maintaining trust. The use of predictive analytics in this context not only safeguards financial assets but also enhances the overall customer experience, establishing a secure and reliable banking environment.
PRO TIP: Some of the largest financial service providers offer solutions for credit fraud prediction and management:
- Accertify, an American Express company, offers end-to-end fraud detection solutions, including customer authentication, SCA optimization, and chargeback management.
- Ekata by Mastercard offers worldwide identity verification and fraud prevention services including a series of APIs built for enterprises and fraud analysts.
- Cybersource, powered by Visa’s Decision Manager software, offers modules to help finance professionals address payment fraud along with ML modules to help merchants grow their reach, with capabilities for currency conversion, global tax compliance, and customer lifetime management.
Conclusion
Predictive analytics in finance has proven to be a game-changer, offering a wealth of opportunities for enhanced decision-making, risk management, and customer engagement. By leveraging advanced statistical models and machine learning algorithms, financial institutions can not only predict future market trends but also develop strategies that align with evolving economic landscapes. As the financial sector continues to embrace these technologies, predictive analytics will undoubtedly play a pivotal role in shaping the future of finance, driving innovation, and fostering a more dynamic and resilient industry.
If you’re looking for a partner to help you develop a custom solution, consider our financial software development services. Our team will partner with you to build a comprehensive predictive analytics strategy. We'll assist you in selecting the most suitable technologies, seamlessly integrating them into your existing tech stack, and delivering a robust, scalable solution.